Using Data analytics, marketers can map customer behavior and develop more personalized experiences for them. This can include carefully crafted in-store displays and email campaigns. They can also create new products and services based on the insights they have. Data analysis can also help businesses identify inefficiencies. It can even uncover mechanical failures that can be used to improve workflows.
Data collection
The most fundamental requirement for data analytics is the collection of data. Data collection is critical in the process of making predictions, forecasts, and managing operations. Accurate data collection ensures that the data is accurate and defined properly. It also gives a baseline to work from, and indicates areas of improvement. Ultimately, data analytics can help a business improve their products and services. It can also help a company build trust with customers and investors. Data collection is a key element in the success of any business, and this process will help you understand what your customers are looking for and what they want.
As more companies begin using data analytics, more companies are collecting data to use for marketing purposes. This data is often contextualized and enables companies to learn more about what their customers are looking for. With this information, companies can improve their marketing campaigns and understand what consumers really want. As marketing becomes more personalized, companies with the right data are standing to benefit the most. A new industry is also emerging alongside big data: data brokers. These firms specialize in collecting and selling customer information.
Data integrity is another fundamental aspect of data collection. Errors in data collection can have a variety of effects, ranging from minor to severe. They can be random, intentional, or systematic.
Data cleaning
Data cleaning is an important part of data analytics, but it can be tedious and annoying. The key is to follow a consistent process to avoid errors and produce accurate results. There are a number of automated data cleaning tools that can help with this process. Scripts can also be created using Excel or other programming languages.
Once the data is clean, it can then be analyzed. This process involves removing outliers from the data that could have an impact on the results. This process can help you develop a better customer segmentation model and send customers more relevant information.
Another step in data cleaning is data mapping. This will help you visualize how your data fits together and can help you monitor errors and corrupt data. By utilizing data mapping, you can improve your business processes and speed up decision-making. The data mapping process can also help you understand what types of errors you have and what you can do to fix them.
Data cleaning is a crucial part of data analytics. The best results come from data that is clean and standardized. Data cleaning involves removing duplicate records, wrong data formats, and missing data. Incorrect data can create errors in the results and make the algorithms unreliable.
Data exploration
Data exploration is an important aspect of data analytics, and it can help you find patterns and trends that otherwise would be difficult to uncover. It also helps you save time and effort by providing clear paths to better analyze data. Data exploration tools are available on the cloud. Some tools used in data exploration are histograms, box plots, and scatter plots. Each one is useful in determining the relationship between two variables. Box plots help you find out the skew and how often values change, while scatter plots help you determine the nonlinearity of a relationship. Other tools for data exploration include correlation heat maps, which show the relationship between two types of data, such as categorical data and numeric data.
Data exploration helps businesses identify areas to focus on. It also helps them make informed decisions about which data to use and which to discard. This allows businesses to focus on the most relevant information and decrease the time spent on analysis that does not yield the best results. These tools can help you analyze a large amount of data with ease.
Data exploration has applications in many different industries. These can range from individual paper research to news aggregation, marketing, finance, and real estate. It can also be used for lead generation. For example, companies can use data mining techniques to extract information from Yellowpages or use web scraping templates to generate leads. Furthermore, content aggregation sites can use data exploration to get data from multiple sources on a regular basis.
Data analysis
Data analysis is a powerful business tool that helps companies meet a variety of goals. However, it’s important to define the objectives of the study. First, identify the problem and purpose of the study. Second, define the type of data to be analyzed. Lastly, interpret the findings. This article will discuss some of the different types of data analysis.
Typically, two types of data analysis are conducted: quantitative and qualitative. Qualitative data is descriptive and gathered from user interviews, surveys, and observations. Quantitative data, on the other hand, is measurable and countable. It aims to answer questions regarding the quantity or average of a variable. Statistical analysis helps to uncover patterns within data.
Data analysis can help a business understand and improve performance. It helps managers make better decisions, solve problems, and create more effective strategies. It helps companies learn about competitors, understand their markets, and tailor their products or services to match consumer needs. Data analysis can also help them determine what type of pricing strategy will be most effective.
Data analysis is a process that allows users to sift through massive amounts of data to derive useful insights. It is critical to gather the right data before starting the analysis.
Cost of data analysis
Cost of data analysis has declined, but not in the way that you might think. Today’s applications can perform many different statistical tests with the click of a button. Additionally, the majority of applications have built-in support for multiple testing, which makes it much easier to carry out statistical tests for a larger dataset.
Data analysis can help you to make better decisions and understand your data better. This process involves gathering and analyzing data, and identifying patterns. You also need to decide what type of analysis you need, and why you need to do it.
Hiring in-house analysts is an option, but it’s a big commitment. You’ll need to hire at least a couple of analysts and pay them a reasonable salary. Even then, they’re not cheap. And you need to make sure that they’re capable of interpreting the data.
The complexity of data analysis will depend on the type of project and the questions you’re asking. In general, however, most monitoring projects will have enough functionality to handle data analysis themselves. For example, you can run an Excel spreadsheet to calculate variables such as length of stay, income, and other variables.
IoT devices as a source of data
IoT devices generate large amounts of data that can be analyzed in real time. This data allows organizations to make real-time decisions about the health of their business operations. For example, e-commerce companies can use IoT data to identify trends in consumer behavior and create targeted content and advertising. In other applications, such as driverless cars, data from IoT devices is critical for the immediate decision-making process.
IoT technology can also help retailers understand customer behavior and spot underperforming areas of their business. The data collected from IoT devices can be used to improve store layouts, display advertisements in remote areas, and so on. However, using IoT data analytics can pose challenges and risks, and organizations should be aware of them. For one thing, it can be costly, and there are privacy and security concerns.
The volume of IoT data is growing exponentially. This data can be anything from CCTV footage to sensor readings. It is important to understand and manage this data to derive value from it. In addition, IoT-generated data can also be used to identify problems or monitor the health of people.
Data analytics software allows businesses to make informed decisions using this data. The resulting analytics can improve business decisions by providing actionable insights. It can also improve safety and surveillance.
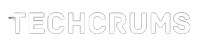